Multimodal
We are now tackling a new interdisciplinary filed of natural language processing and computer vision. We believe NLP and CV can both help each other to realize higher-level intelligence and applications.
We are also collaborating with Perception and Language Understanding (PLU) Group at AIST.
Zero-shot machine translation using multimedia pivot
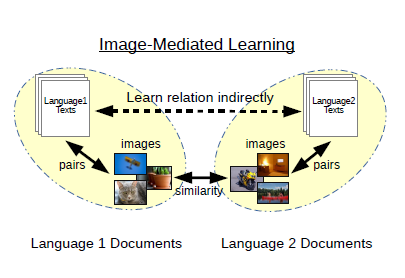
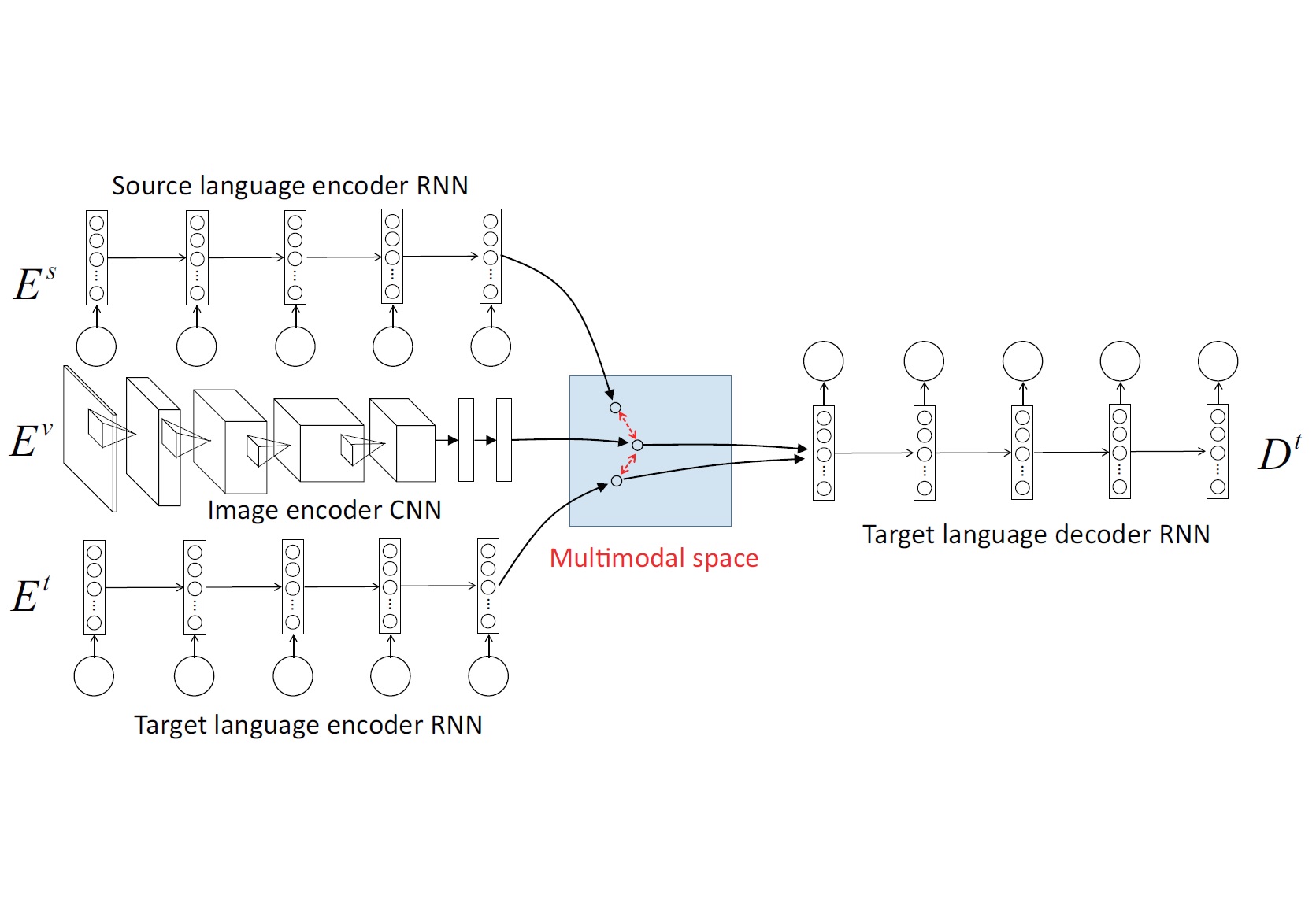
To train a machine translation system, we usually require a parallel corpus consisting of example documents written in two (i.e., source and target) languages. However, preparing parallel documents is not very easy because most of the generic documents are monolingual. Meanwhile, today, we can find many document in Web accompanied by relevant images such as posts on blogs or SNS. In this work, we propose a method to train a machine translation system with no direct parallel data by using the images as "pivot" to indirectly align source and target languages.
- Hideki Nakayama, Noriki Nishida, "Zero-resource Machine Translation by Multimodal Encoder-decoder Network with Multimedia Pivot", Machine Translation, Volume 31, Issue 1-2, pp.49-64, 2017. pdf
- Ruka Funaki, Hideki Nakayama, "Image-mediated learning for zero-shot cross-lingual document retrieval", Empirical Methods in Natural Language Processing (EMNLP), 2015. pdf
- Pascal1K+jp dataset
Automatic video captioning
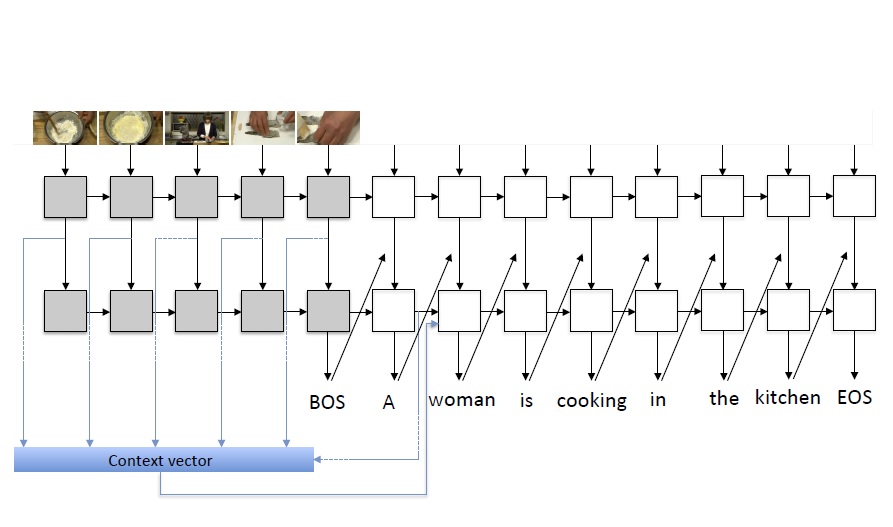
Automatic video description generation has recently been getting attention after rapid advancement in image caption generation. Automatically generating description for a video is more challenging than for an image due to its temporal dynamics of frames. Most of the work relied on Recurrent Neural Network (RNN) and recently attentional mechanisms have also been applied to make the model learn to focus on some frames of the video while generating each word in a describing sentence. In this paper, we focus on a sequence-to-sequence approach with temporal attention mechanism. We analyze and compare the results from different attention model configuration.
- Natsuda Laokulrat, Naoaki Okazaki, Hideki Nakayama, "Incorporating Semantic Attention in Video Description Generation", In Proceedings of LREC, pp.3011-3017, 2018. pdf
- Natsuda Laokulrat, Sang Phan, Noriki Nishida, Raphael Shu, Yo Ehara, Naoaki Okazaki, Yusuke Miyao, Shin'ichi Satoh, and Hideki Nakayama, "Generating Video Description using Sequence-to-sequence Model with Temporal Attention", International Conference on Computational Linguistics (COLING), 2016. pdf