Machine learning
Architecture design and initialization of convolutional neural networks
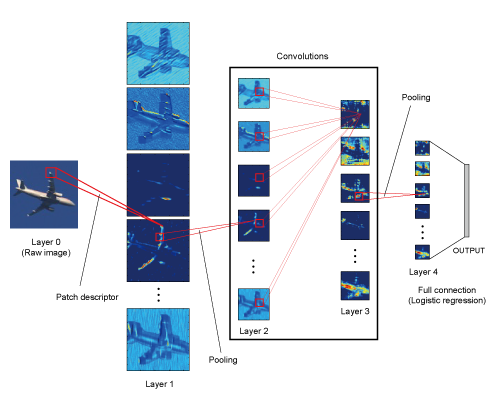
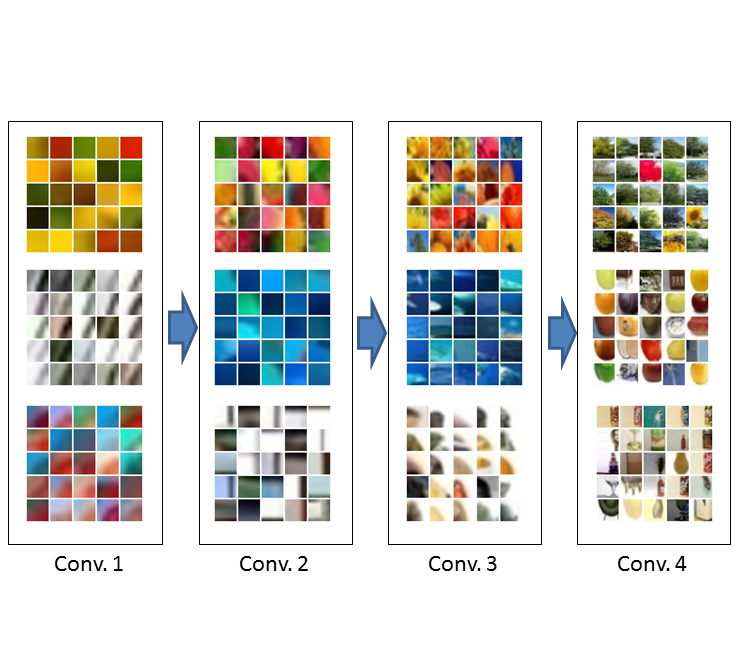
Deep learning is a recently re-established framework where huge artificial neural networks are directly applied to raw data and optimized to model the best architecture. Although it has achieved surprisingly high performance in many areas, there are still many drawbacks. For example, it is known to require huge computational cost and human tuning. In our lab, we are developing a fast and analytic learning method of convolutional neural networks that achieves promising results on some benchmarks.
- Hideki Nakayama, "Efficient discriminative convolution using Fisher weight map", In Proceedings of British Machine Vision Conference (BMVC), 2013. pdf
Frequency-domain convolution
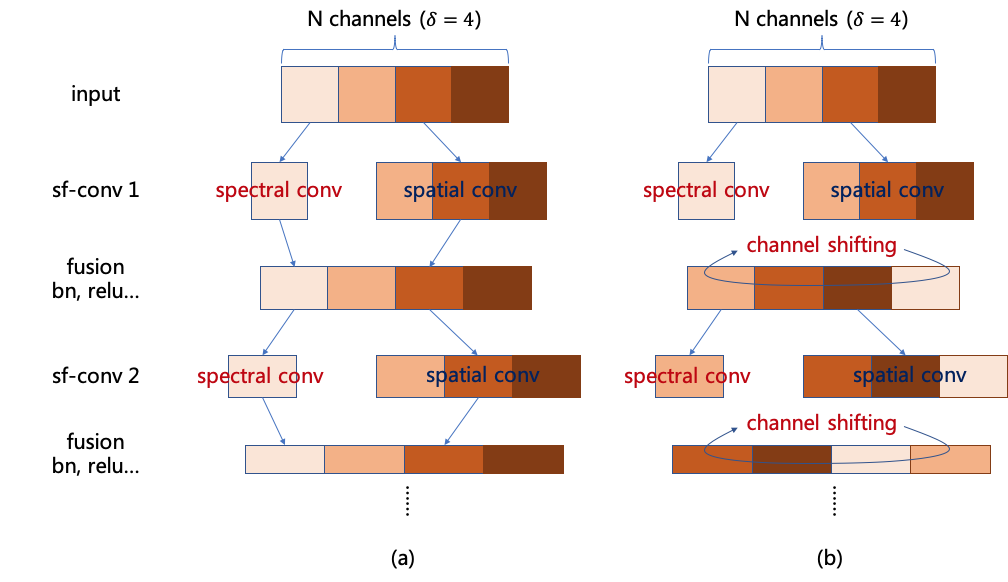
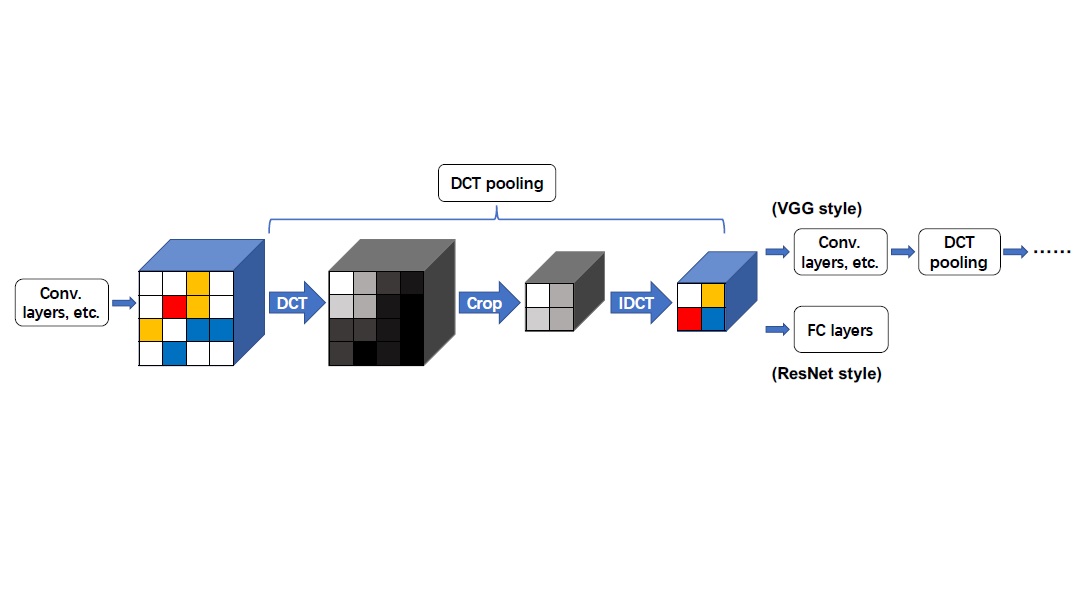
Deep convolutional neural networks (CNNs) extract local features and learn spatial representations via convolutions in the spatial domain. Beyond the spatial information, some works also manage to capture the spectral information in the frequency domain by domain switching methods like discrete Fourier transform (DFT) and discrete cosine transform (DCT), which are known to achieve much faster convolution operations. We are developping novel DCT-based architectures to realize compact and fast CNN models.
- Yuhao Xu and Hideki Nakayama, "Shifted Spatial-Spectral Convolution for Deep Neural Networks", In Proceedings of the 1st ACM International Conference on Multimedia in Asia (MM Asia), 2019.
- Yuhao Xu, Hideki Nakayama, "DCT Based Information-Preserving Pooling for Deep Neural Networks", In Proceedings of the 26th IEEE International Conference on Image Processing (ICIP), 2019.
Multi-label classification model
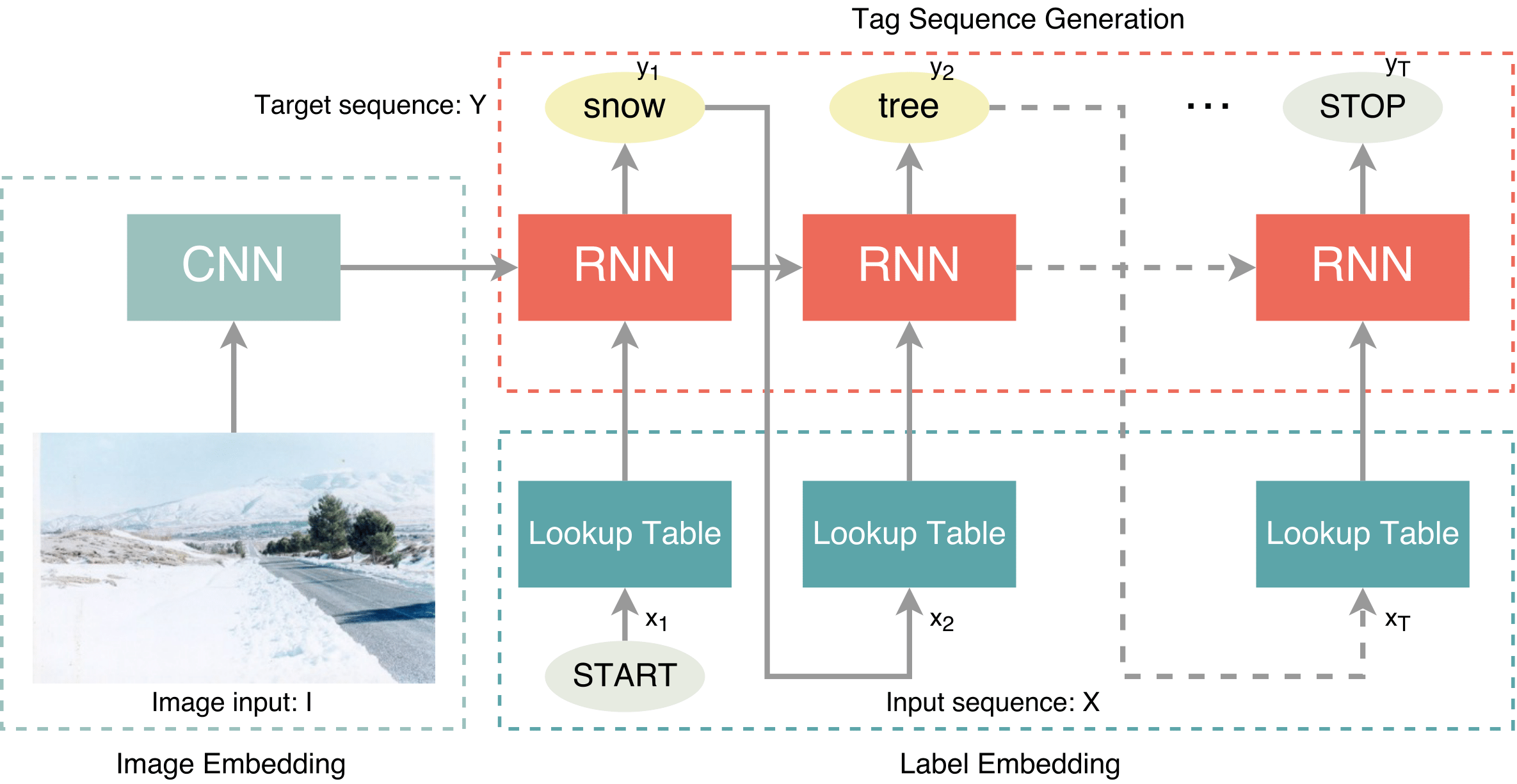
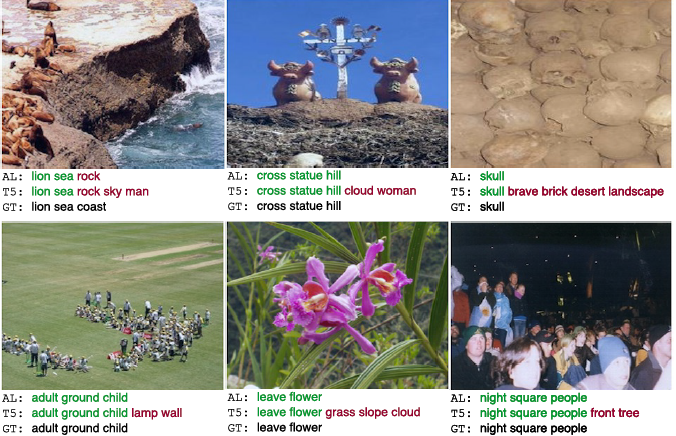
Annotating multiple related keywords to images is essential for managing and retrieving the large scale images available on the web. However, in contrast to the intuition and the fact that different images usually contain various amounts of information, previous researches simply adopt a fixed number of keywords for all the images. This conventional setting makes comparing research results easy, but it has a negative effect to the annotation results. To solve this problem, we have developed a novel approach to predict an appropriate number of keywords according to the specific image contents. Meanwhile, our experiments indicate that different orders for the keyword prediction procedure has a huge impact on the annotation results. A web demo is also available for this project: Recurrent Image Annotator.
- Jiren Jin, Hideki Nakayama, "Annotation Order Matters: Recurrent Image Annotator for Arbitrary Length Image Tagging", International Conference on Pattern Recognition (ICPR), 2016. pdf
Compressing representations by neural networks
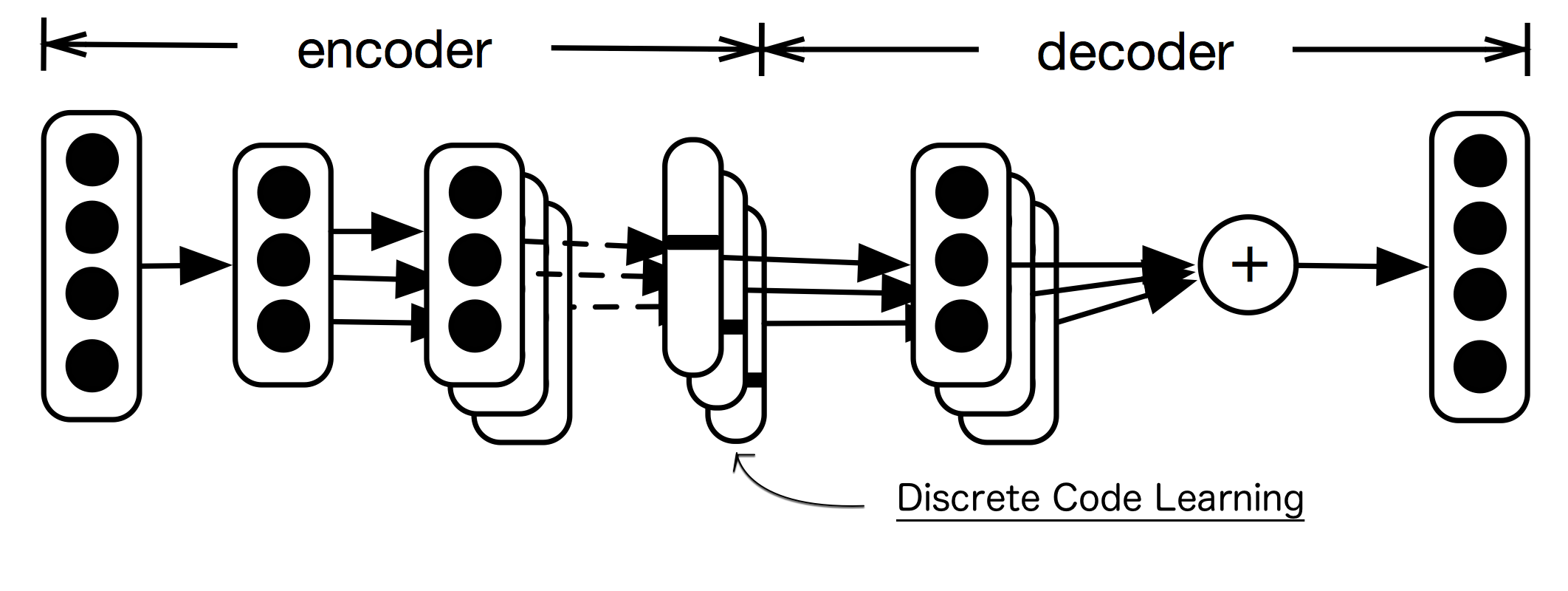
Natural language processing (NLP) models often require a massive number of parameters for word embeddings, resulting in a large storage or memory footprint. Deploying neural NLP models to mobile devices requires compressing the wordembeddings without any significant sacrifices in performance. For this purpose,we propose to construct the embeddings with few basis vectors. For each word, the composition of basis vectors is determined by a hash code. To maximizethe compression rate, we adopt the multi-codebook quantization approach insteadof binary coding scheme. Each code is composed of multiple discrete numbers,such as (3,2,1,8), where the value of each component is limited to a fixed range. We propose to directly learn the discrete codes in an end-to-end neural networkby applying the Gumbel-softmax trick. Experiments show the compression rateachieves 98% in a sentiment analysis task and 94%∼99% in machine translationtasks without performance loss.
- Raphael Shu and Hideki Nakayama, "Compressing Word Embeddings via Deep Compositional Code Learning", International Conference on Learning Representations (ICLR), 2018. pdf
Multimodal learning
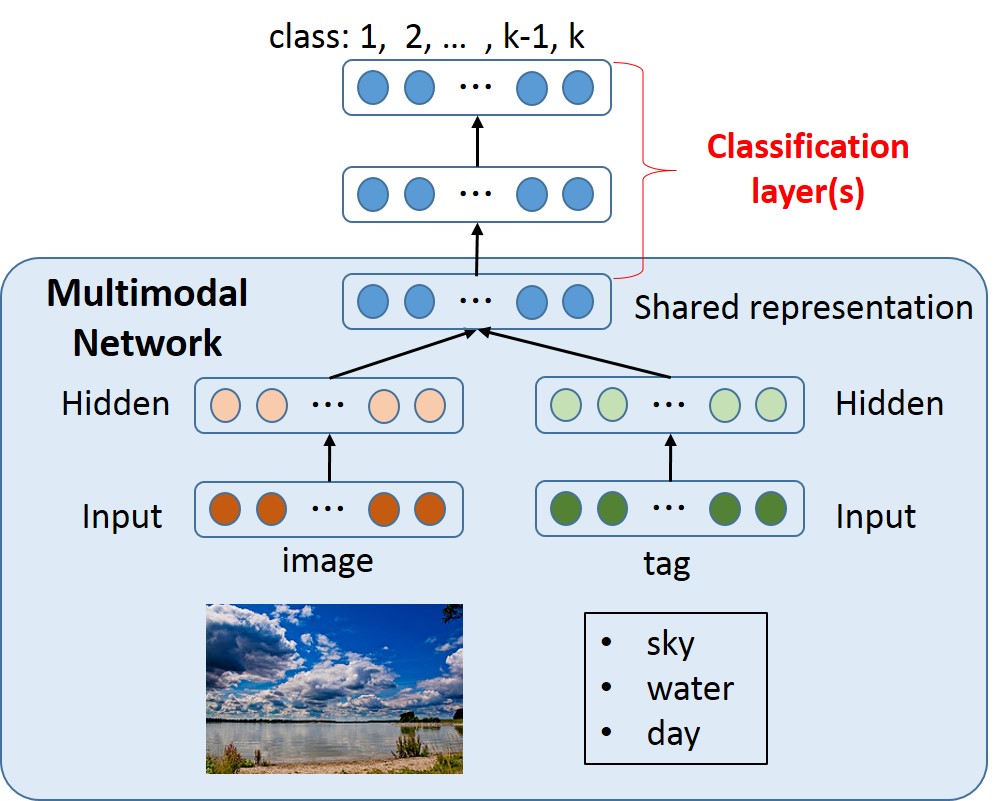
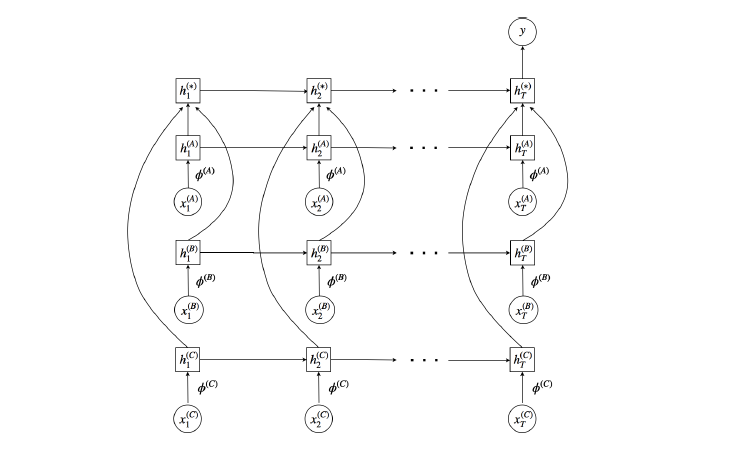
In recent years, it has become possible to get many different types of information from various sensors. Combinig such multi-modal information may help us to increase the accuracy and robustness of recognition, which is called "Multi-modal learning". We are developing deep models in various aspects to flexibly handle such multi-modal information.
- Noriki Nishida, Hideki Nakayama, "Multimodal gesture recognition using multi-stream recurrent neural network", Pacific-Rim Symposium on Image and Video Technology (PSIVT), 2015. pdf